machine learning features meaning
The main logic in machine learning for doing so is to present your learning algorithm with data that it is better able to regress or classify. Height Sex Age 615 M 20 555 F 30 645 M 41 555 F 51.
Machine Learning Life Cycle Datarobot Artificial Intelligence Wiki
As others have pointed out latent means not directly observable.
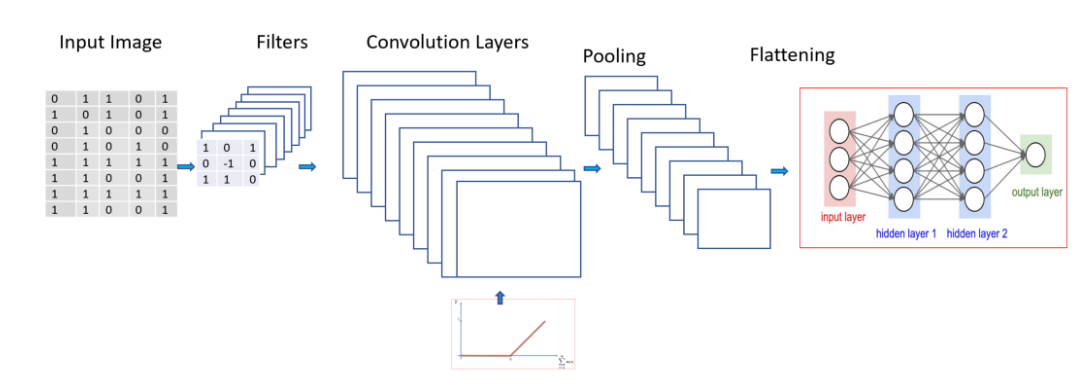
. Hand-crafted features refers to derived variablescovariatesfeatures. ML is one of the most exciting technologies that one would have ever come across. Answer 1 of 4.
Machine learning is a branch of artificial intelligence AI and computer science which focuses on the use of data and algorithms to imitate the way that humans learn gradually improving its accuracy. If feature engineering is done correctly it increases the. The label could be the future price of wheat the kind of animal shown in a picture the meaning of an audio clip or just about anything.
Or you can say a column name in your training dataset. IBM has a rich history with machine learning. Its up to data and algorithm to define their value.
In machine learning and pattern recognition a feature is an individual measurable property or characteristic of a phenomenon. Eg the cat-detecting neuron feature in Googles image recognizer a few years ago. Feature engineering is the pre-processing step of machine learning which is used to transform raw data into features that can be used for creating a predictive model using Machine learning or statistical Modelling.
Similarly for another dataset consisting of handwritten digits the latent variables may be angle of edges skew etc. Learned features are those that automatically emerge from complex models. Feature Engineering for Machine Learning.
A feature is an input variablethe x variable in simple linear regression. One popular option is to replace missing data with -99999. Machine Learning is the field of study that gives computers the capability to learn without being explicitly programmed.
What are features in machine learning. The Features of a Proper High-Quality Dataset in Machine Learning A good dataset combines high quality with sufficient quantity However before you decide on what sources to use while collecting a dataset for your ML model consider the following features of a good dataset. Eg the MFCCs of an audio signal for speech recognition.
In our dataset age had 55 unique values and this caused the algorithm to think that it was the most important feature. Prediction models use features to make predictions. It learns from them and optimizes itself as it goes.
In the real world many data sets are very messy. A feature map is a function which maps a data vector to feature space. With many machine learning classifiers this will just be recognized and treated as an outlier feature.
Data mining techniques employ complex algorithms themselves and can help to provide better organized data sets for the machine learning application to use. This is because the feature importance method of random forest favors features that have high cardinality. In datasets features appear as columns.
Machine learning looks at patterns and correlations. Forgetting to use a feature scaling technique before any kind of model like K-means or DBSCAN can be fatal and completely bias. Feature engineering in machine learning aims to improve the performance of models.
Feature engineering is the process of using domain knowledge of the data to create features that make machine learning algorithms work. Feature importances form a critical part of machine learning interpretation and explainability. A feature is a measurable property of the object youre trying to analyze.
Data mining is used as an information source for machine learning. Last Updated. When approaching almost any unsupervised learning problem any problem where we are looking to cluster or segment our data points feature scaling is a fundamental step in order to asure we get the expected results.
The image above contains a snippet of data from a public dataset with information about passengers on the ill-fated Titanic maiden voyage. Features are nothing but the independent variables in machine learning models. A simple machine learning project might use a single feature while a more sophisticated machine learning project could.
The goal of this process is for the model to learn a pattern or mapping between these inputs and the target variable so that given new data where the target is unknown the model can accurately predict the target variable. In Machine Learning feature means property of your training data. Feature engineering is the process that takes raw data and transforms it into features that can be used to create a predictive model using machine learning or statistical modeling such as deep learning.
Features are usually numeric but structural features such as strings and graphs are used in. For a movie its latent features determine the amount of action romance story-line a famous actor etc. What is a Feature Variable in Machine Learning.
The ability to learn. In other words latent variables are like step that bridges the gap between your observed variables and the desired prediction. Suppose this is your training dataset.
The aim of feature engineering is to prepare an input data set that best fits the machine learning algorithm as well as to. Quality of a Dataset. As it is evident from the name it gives the computer that makes it more similar to humans.
What is required to be learned in any specific machine learning problem is a set of these features independent variables coefficients of these features and parameters for coming up with appropriate functions or models also termed as. Then here Height Sex and Age are the features. Latent variables allow to render the models more powerful in terms what can be modeled.
You can also just drop all featurelabel sets that contain missing data but then youre maybe leaving a lot of data out. Features are individual independent variables that act as the input in your system. New features can also be obtained from old features.
A machine learning model maps a set of data inputs known as features to a predictor or target variable. Choosing informative discriminating and independent features is a crucial element of effective algorithms in pattern recognition classification and regression.
Ensemble Feature Selection In Machine Learning By Optimalflow Machine Learning Machine Learning Models The Selection
Feature Selection Techniques In Machine Learning Javatpoint
Feature Selection Techniques In Machine Learning Javatpoint
Feature Selection Techniques In Machine Learning Javatpoint
What Are Feature Variables In Machine Learning Datarobot Ai Wiki
What Are Feature Variables In Machine Learning Datarobot Ai Wiki
What Is Machine Learning Definition How It Works Great Learning
What Is A Pipeline In Machine Learning How To Create One By Shashanka M Analytics Vidhya Medium
Convolutional Neural Network Feature Map And Filter Visualization By Renu Khandelwal Towards Data Science
Feature Scaling Standardization Vs Normalization
Feature Vector Brilliant Math Science Wiki
Introduction To Dimensionality Reduction Technique Javatpoint
Supervised Machine Learning Javatpoint
Feature Selection Techniques In Machine Learning Javatpoint
A Tour Of Machine Learning Algorithms
Feature Vector Brilliant Math Science Wiki
Convolutional Neural Network Feature Map And Filter Visualization By Renu Khandelwal Towards Data Science